Machine Learning in Healthcare: Revolutionizing Patient Care
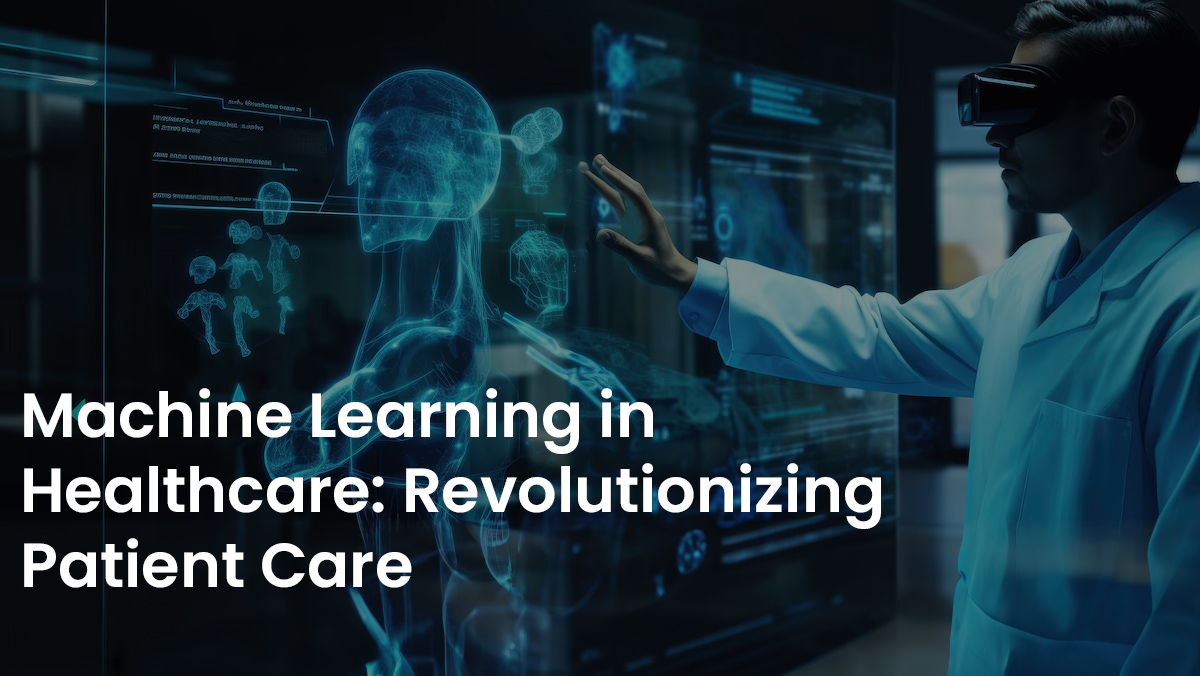
The worth of Machine Learning in Healthcare has been evident in recent times as this data-driven approach changes the medical landscape worldwide.
Truly, Machine Learning in Healthcare enables timely intervention-cum-informed decisions based on advanced analytics, which draw from disparate clinical datasets. It allows practitioners to understand subtle variations, anticipate patient needs, and enhance treatment strategies across multiple specialties.
Such evolution is synonymous with artificial intelligence in medicine, where tools not only interpret this complex data but also support clinical decisions with an accuracy that has never before been achieved.
Policymakers and researchers are, therefore, in favour of these technologies, as reported, on the grounds that they could reform outcomes for many populations of patients and spur new models of care. Most of the Healthcare facilities, now using AI in Healthcare, have benefited in the improvement of outcome or reducing burdens in management.
At the heart lies Predictive Analytics, which gives clinicians the ability to know even the most simple risk factors ahead of the event. Similarly, Healthcare Data Analytics exposes correlations governing evidence-based practices and personalized care.
In conclusion, Machine Learning in Healthcare proves to be central to future medicine as the increasingly digital innovations penetrate health systems. The fusion of technology and clinical expertise promises to address escalating costs and resource constraints and contribute to more efficient, patient-centered models of care.
The Power of Data and ML in Modern Healthcare
Machine Learning in healthcare is powerful enough to analyze enormous amounts of clinical data with fantastic speed. Hospitals acquire learning from insights, which guide preventive measures and intervention. It is this reliance on data that has shaped the Healthcare Data Analysis shaping personalized care plan.
In the same vein, AI in Healthcare has evolved into a risk assessment capable of identifying complications on the rise. Other points raised by the researchers were the continuous revision of models as fresh data from the patient improves the algorithm. They have a learning environment that contributes to developing even better predictive tools from each clinical encounter.
By using Artificial Intelligence in Medicine, experts are brought together by real-time decision-making across the diagnostic and therapeutic divide. As Predictive Analytics in Healthcare becomes even more robust, it opens the doors toward accurate prognostics on disease progression and their impact on resource allocation-hence, improving outcomes.
Notably, Machine Learning in Healthcare increases efficiency because tasks are automated and such face-to-face patient interactions become even more frequent. These future technologies promise both speedier and more accurate screening results and a proactive approach.
Embracing Machine Learning in Healthcare systems saves healthcare costs, minimizes errors, and ensures big interventions take place when most needed, thereby improving the delivery of care.
Enhancing Diagnostics with ML-Powered Systems
One of the transformative prospects of Machine Learning in Healthcare is in diagnostic imaging. Using advanced algorithms to analyze radiological scans and images, clinicians pinpoint anomalies with utmost precision, thereby dampening the rate of misdiagnoses.
This is one example of how Predictive Analytics in Healthcare can assist the doctors in reaching variable conclusions, improving accuracy and speed in critical diagnostic processes. Apart from that, AI in Healthcare allows instant access to specialists’ insights in real-time environments, particularly oncology, where early intervention can be a lifesaver.
Meanwhile, Healthcare Data Analysis drives continuous model refinements by pooling information from various healthcare institutions so that each dataset enhances the overall knowledge pool. Patients benefit from appropriate and timely treatment recommendations, thanks to well-established image-recognition tools supported by Artificial Intelligence in Medicine.
Hence, Machine Learning in Healthcare enables personalized care pathways allowing physicians to pinpoint the precise stage and nature of medical conditions. This transition toward data-led diagnostics embodies a new patient-care paradigm whereby algorithms assist health care providers in elucidating a deluge of clinical data.
As these tools make headway to mass deployment, scaling down false positives, minimizing invasive procedures, and fast-tracking treatment protocols represent the most stellar advantage of Machine Learning in Health Kin Solutions globally.
Predictive Analytics and Early Disease Detection
Apart from imaging, Machine Learning in Healthcare provides a strong motive for early disease detection. The electronic health records, laboratory findings, and genetic markers of a patient can be examined to enable the practitioner to foresee medical roadblocks. Such advanced prognosticating nearly qualifies as Predictive Analytics in Healthcare, wherein algorithms conduct painstaking data pattern analyses that bring forth early warning signs to decrease serious complications.
On the other hand, AI in Healthcare devices worn on the body and mobile applications are collecting real-time health metrics: data which practitioners may use to develop tailored individual preventive measures. In this way, Healthcare Data Analysis affords a holistic view of a patient’s well-being, creating windows for intervention as early as possible.
This could refer to the function of Artificial Intelligence in Medicine, which especially comes in handy when upgrading risk stratification methods in this consideration since it could help the teams in Healthcare prioritize patients who actually need prompt attention.
Through Machine Learning in Healthcare, caregivers transform reactive care models into proactive paradigms dealing with wellness preservation rather than solely disease management. This shift achieves a decrease in hospitalization rates, a drop in treatment costs, and an increase in people empowering themselves over their treatment.
As the medical community increasingly recognizes the significance of early detection, Machine Learning in Healthcare solidifies its place as a primary driver of accessible, efficient, and effective patient outcomes.
Personalized Medicine and Treatment Optimization
A personalized treatment plan is yet another frontier where Machine Learning in Healthcare has cut deep. By analyzing the genetic profile, lifestyle factors, and past medical histories, physicians can create targeted treatments that fit the individual needs of a patient.
Healthcare Data Analysis supports the approach through aggregating the findings from populations similar to the patient being attended to come up with better treatment suggestions. Predictive Analytics in Healthcare builds solid predictive models that can then be used by providers to determine an effective dosage in case of drug interaction or the necessary medication in treatment.
The combination of Artificial Intelligence in Medicine with clinical expertise allows leaving behind simple protocols adopted by all and gaining patients’ better satisfaction as well as improved outcomes. Patients are tracked while in real time. It raises timely adjustments and proactive interventions.
However, Machine Learning in Healthcare brings out optimized workflows with lesser adverse effects and surely streamlined resource allocation-all these with patient orientation. Indeed, such data-driven strategies in health care would easily accumulate more human-environment interactions for improving hospital capacity management and avoiding overcrowding and waiting time.
Perhaps Machine Learning in Healthcare’s broadest promise is its ability, as today more institutions are capitalizing on technology, to bring about a paradigm shift from generalization towards meticulous, precise, and scalable care solutions that cover all patient demographics.
Challenges and Ethical Considerations
Despite such exhilarating advancements, these kinds of propositions have many hurdles in implementing Machine Learning in Healthcare. Topmost is making sure of data privacy; also these predications should keep safe confidential patient health information when casting robust predictive models.
Not the least urgent, because of Healthcare Data Analysis, implemented with a great need to secure handling of gigantic data sets from various sources, is still data privacy requirements for health industry. Data loss may also occur in case of, for example, certain health insurance records being confidential evoked by or used in training AI for development purposes.
Bias from the data remains another problem of AI in Healthcare, as such training data may not be representative. That’s why model inclusivity is very much needed. Moreover, industry champions are well aware of the aspect of regulatory frameworks since watchdogs will try to attain a middle ground between innovativeness and ethics.
Under this, Artificial Intelligence in Medicine should be transparent and fair, especially when reaching life-changing decisions such as treatment recommendations. Further issues include the consent of the patient regarding the use of Predictive Analytics in Healthcare and whether the reach would spill over into an important aspect of the patient monitoring.
Nevertheless, the advantages of Machine Learning in Healthcare far outweigh its cons, provided clear-cut ethical standards are established by all stakeholders concerned.
In dismantling these challenges, the medical fraternity would be able to unleash the full potential of Machine Learning in Healthcare to effect an unbiased, equable delivery of care.
The Future of ML in Healthcare
Looking ahead, the future of Machine Learning in Healthcare is poised to encompass increasingly sophisticated applications. From robotic-assisted surgeries to virtual nursing assistants, AI in Healthcare is rapidly expanding, reshaping how patients receive care in real-time settings.
Advances in Artificial Intelligence in Medicine also hint at the possibility of more refined drug discovery, leveraging data from genomics and clinical trials to develop treatments with greater efficacy.
Meanwhile, Predictive Analytics in Healthcare continues to evolve, enabling organizations to model public health risks, track disease outbreaks, and streamline emergency responses.
Coupled with Healthcare Data Analysis, these capabilities create a unified ecosystem where patient data seamlessly informs both immediate interventions and long-term healthcare strategies.
In tandem with these developments, Machine Learning in Healthcare is intersecting with telemedicine platforms, ensuring patients can access specialists regardless of geographical barriers. This intersection likewise paves the way for more integrative patient monitoring solutions, as wearable devices capture continuous physiological data.
As the technology matures, Machine Learning in Healthcare stands ready to pioneer breakthroughs that could revolutionize how clinicians diagnose, treat, and manage diseases across diverse populations. Ultimately, the potential to optimize outcomes and reduce costs places these AI-driven tools at the forefront of modern medicine.
Interdisciplinary Collaboration and Integration
Collaboration between clinicians, data scientists, and technology experts becomes crucial as AI-driven solutions get more and more integrated into clinical workflows.
The marriage of practical understanding with the technical intricacies of Healthcare Machine Learning will enable care teams to design solutions that will perfectly fit into the given protocols.
Predictive Analytics in Healthcare is expected to play an even more central role in this context with advanced forecasting methods further carving out refined allocations for resources and patient engagement strategies.
In the bid to enrich the field, lots of hospitals are now using AI in Healthcare to advance diagnostic accuracy and hasten administrative tasks, while simultaneously letting medical practitioners focus more on personalized services. In them lies a common future where clinical knowledge and artificial intelligence in medicine stand side by side, enhancing patient outcomes.
Growth in Healthcare Data Analytics underpins the increasing significance of well-guarded, interoperative frameworks. As Machine Learning in Healthcare transcends most health systems, particularly those found in remote areas, questions stand right if when the proposed models could be applied.
This would only make the evidence-based and learning curve observed much clearer for embedding integrated care approaches.
Thus, for successful use of Machine Learning in Healthcare, very clear benchmarking and effective interdisciplinary dialogues remain very important for healthcare establishments..
Conclusion
Finally, the advent of Machine Learning in this field opens up a whole new possibility for developing precise, proactive, and patient-focused care that will soon become the global norm. This Predictive Analytics in Healthcare can push records forward by initiating or delaying a complication so that treatment may have far better results.
Along with this development, AI in healthcare also takes clinical workflow velocity to research innovations and critical decision-making in hospitals and research centers and beyond. Also, the analysis of healthcare data into big data knows most of the patterns that further strengthens itself as a pillar of artificial intelligence in medicine for a much-evolving modern-day healthcare.
As hospitals and medical centers come to adopt Machine Learning in Health Systems into the everyday way of doing things, the greater chances of having better quality care are seen. But to fully realize the promise of the breakthroughs, they need to be partnered by other organizations with substantial experience in designing and implementing robust solutions.
At FX31 Labs, we provide a software dedicated team to guide healthcare providers through every step of this digital transformation. Innovative machine learning development services and AI integration services build walls between all leaders and awkward patients of the demarcated yet thriving industry.
Thus, leaders define a medical future directed toward evidence-based care and sustainable innovation through joint investment. In this way, Machine Learning will end by defining new limits of possibly a redefined paradigm of effective treatments, seamless patient experiences, and long-term improvements in population health. It would take a lot of collaboration.