How to Transform your Business with Generative AI Development Services
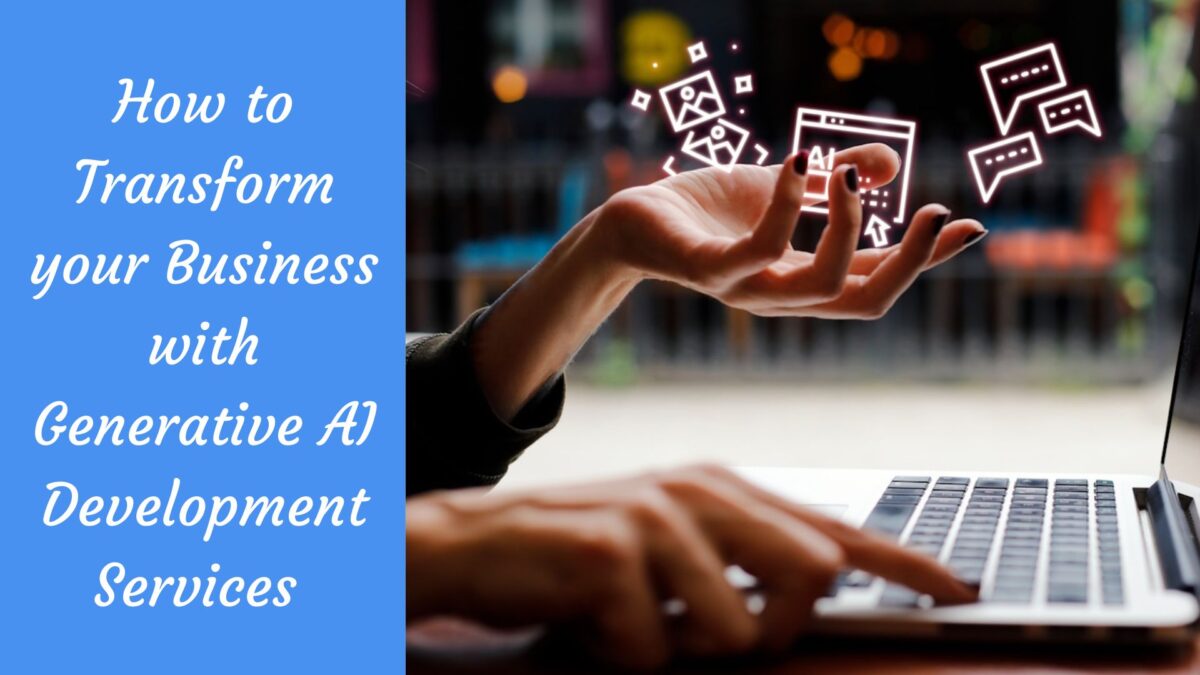
Generative AI development services are transforming the technological landscape by enabling the creation of innovative, high-quality content across various industries.
These services utilise advanced AI techniques to generate new data, such as text, images, and audio, by learning from existing datasets.
This capability not only enhances creativity and efficiency but also opens new possibilities for personalised marketing, medical imaging, and more.
As businesses seek to leverage these cutting-edge technologies, choosing the right generative AI development company becomes crucial for achieving successful and impactful outcomes.
Understanding Generative AI
Generative AI refers to artificial intelligence systems designed to generate new content, such as text, images, audio, and video, by learning patterns from existing data.
Unlike traditional AI models that focus on classification and prediction, generative AI creates original data that mimics the structure and characteristics of the input data.
This capability has revolutionised various industries, enabling advancements in content creation, personalised marketing, medical imaging, and more using gen ai development process.
By leveraging techniques like Generative Adversarial Networks (GANs) and Variational Autoencoders (VAEs), generative AI continues to push the boundaries of innovation and creativity.
Core Components of Generative AI
Generative AI Models
Generative AI models are central to modern AI systems. These models generate new data based on patterns they learn from existing datasets.
For example, a generative AI model can create realistic images, write articles, or even compose music by analysing large amounts of existing data and identifying underlying structures.
There are several types of generative AI models, including Generative Adversarial Networks (GANs), Variational Autoencoders (VAEs), and Transformer-based models. Each type has its strengths and applications.
GANs, for instance, are widely used for image generation, creating visuals that are almost indistinguishable from real photographs.
VAEs are often used in scenarios that require the generation of new data points while maintaining a continuous latent space, which is crucial for tasks like anomaly detection and creative content generation.
Generative AI solution development focuses on creating and fine-tuning these models to meet specific business needs.
By leveraging advanced algorithms and neural networks, these services develop AI models that enhance various applications, from automated content creation to sophisticated data analysis.
Generative AI Solutions
Generative artificial intelligence solutions encompass a wide range of applications designed to solve specific problems using generative models. These solutions are implemented across different industries, including healthcare, finance, marketing, and entertainment.
In healthcare, generative AI solutions are used for drug discovery and medical imaging. By generating synthetic data, these solutions accelerate research and development processes, making them faster and more cost-effective.
In finance, generative AI helps in fraud detection and risk management by generating scenarios and testing the robustness of financial models.
Marketing professionals use generative AI to create personalised content and advertisements. These solutions analyse customer data to generate targeted marketing campaigns that significantly improve engagement and conversion rates.
Additionally, in the entertainment industry, generative AI is revolutionising content creation, enabling the production of unique and engaging media, such as video games, films, and music.
The development of these solutions involves a deep understanding of the specific requirements of each industry and the ability to adapt generative models to meet these needs. This is where generative AI development services play a crucial role, offering tailored solutions that drive innovation and efficiency.
Natural Language Processing and Its Role
Natural Language Processing (NLP) is a subfield of AI that focuses on the interaction between computers and humans through natural language. NLP is vital for understanding and generating human language, making it an essential component of generative AI.
One key application of language models in generative AI is the creation of chatbots and virtual assistants. These tools use advanced NLP algorithms to understand and respond to human queries, providing personalised and context-aware responses.
This capability is particularly valuable in customer service, where AI-powered virtual assistants can handle a significant volume of interactions, improving efficiency and customer satisfaction.
NLP also plays a crucial role in content generation. Generative AI models use NLP to create coherent and contextually relevant text, which can be used in various applications, from automated news articles to creative writing.
Additionally, sentiment analysis, a technique used to understand and classify emotions in text, relies heavily on NLP. This analysis helps businesses gauge public sentiment about their products or services, enabling better decision-making and strategy development.
Generative AI development services incorporate NLP techniques to enhance the functionality and effectiveness of AI solutions. By combining NLP with generative models, these services create more intuitive and human-like interactions, making AI applications more accessible and user-friendly.
Benefits of Generative AI Development Services
Enhancing Business Processes
Generative AI development services can significantly enhance business processes. By leveraging advanced AI models and machine learning algorithms, businesses can automate routine tasks, streamline operations, and improve overall efficiency and fulfil business objectives.
For instance, companies can use generative AI to analyse existing data and predict market trends, helping them make informed decisions. This technology can also optimise supply chain management by predicting demand and managing inventory more effectively.
Moreover, generative AI can transform content creation processes. Businesses can generate high-quality content, such as reports, articles, and marketing materials, with minimal human intervention.
This not only saves time but also ensures consistency and accuracy. For example, AI-powered tools can create personalised marketing campaigns that engage customers more effectively, driving higher conversion rates and customer satisfaction.
Improving Customer Satisfaction
Generative AI development services play a crucial role in enhancing customer satisfaction. AI-powered virtual assistants and chatbots, driven by natural language processing (NLP), provide instant support and personalised responses to customer queries.
This 24/7 availability ensures that customers receive timely assistance, improving their overall experience.
Furthermore, generative AI models can analyse customer feedback and sentiment to understand their needs and preferences better.
This insight allows businesses to tailor their products and services to meet customer expectations more effectively. For instance, AI can identify common issues in customer feedback and suggest improvements, leading to better product development and increased customer loyalty.
Another application of generative AI in customer satisfaction is in creating personalised content. By analysing customer data, AI can generate targeted advertisements and recommendations, making customers feel valued and understood.
This personalisation enhances customer engagement and fosters long-term relationships.
Enabling Advanced AI Models and Solutions
Generative AI development services enable businesses to harness advanced AI models and solutions. These services involve developing and fine-tuning AI models, such as Generative Adversarial Networks (GANs) and Variational Autoencoders (VAEs), to address specific business needs.
For example, GANs are widely used for image generation, creating realistic visuals for marketing and entertainment purposes.
In the healthcare sector, generative AI solutions are used for drug discovery and medical imaging. By generating synthetic data, these solutions accelerate research and reduce costs.
In finance, generative AI helps detect fraud and manage risk by simulating various scenarios and testing the robustness of financial models.
Types of Generative AI Models and Their Applications
AI Models and Their Uses
Generative AI models are powerful tools that create new content by learning patterns from existing data. These models have a wide range of applications across various industries:
Entertainment:
Generative AI models produce realistic images and videos, enhancing visual media quality and creativity.
Marketing:
These models generate personalised advertisements and content, improving engagement and conversion rates.
Healthcare:
Generative AI models develop synthetic medical data to aid in research and diagnosis, providing realistic simulations that help understand complex medical conditions.
Generative AI models are indispensable in today’s technological landscape due to their versatility and wide applications.
Machine Learning Models in Generative AI
Machine learning models are crucial in generative AI development services. These models, like Generative Adversarial Networks (GANs) and Variational Autoencoders (VAEs), learn from data to generate new, original content:
Generative Adversarial Networks (GANs):
GANs consist of two neural networks – a generator and a discriminator – that work together to produce high-quality synthetic data. The generator creates new data samples, while the discriminator evaluates them, leading to continuous improvement.
Variational Autoencoders (VAEs):
VAEs create new data points in a continuous latent space, making them ideal for image synthesis and anomaly detection.
In finance, these models generate scenarios for risk assessment and fraud detection, helping organisations make informed decisions and enhance security.
Neural Networks and Their Importance
Neural networks are the backbone of generative AI models, providing the computational power needed to process vast amounts of data and generate new content. These networks mimic the human brain’s structure, with layers of interconnected nodes that work together to analyse and interpret data.
In generative AI, neural networks create models that generate realistic and high-quality content, such as images, text, and audio. Their importance in generative AI includes:
High Accuracy and Creativity:
Neural networks enable the creation of sophisticated models that learn and adapt to new data.
Advanced AI Systems:
In the automotive industry, neural networks develop AI systems for autonomous vehicles, allowing them to navigate complex environments and make real-time decisions.
Generative AI development services leverage neural networks to create custom solutions tailored to specific business needs, achieving greater efficiency, innovation, and competitiveness.
Implementing Generative AI Solutions
Development Services and Their Impact
Generative AI development services provide the foundation for implementing advanced AI solutions. By leveraging these services, businesses can harness the power of AI to automate processes, enhance decision-making, and create innovative products.
For instance, AI models can generate synthetic data, improving the training and evaluation of other AI algorithms. This approach not only saves time and resources but also boosts efficiency and accuracy.
Development services also focus on customising AI solutions to meet specific business needs. This includes fine-tuning AI models, integrating them with existing systems, and ensuring they operate seamlessly within the business environment.
The impact is significant, as businesses can achieve higher productivity, reduced costs, and improved customer satisfaction.
Generative AI Model Replication
One of the critical aspects of generative AI development services is model replication. This involves creating multiple instances of a generative AI model to perform various tasks simultaneously.
For example, in the entertainment industry, generative AI models can produce different versions of a movie scene, each with unique elements, allowing filmmakers to choose the best option.
Model replication also plays a vital role in industries like healthcare and finance. In healthcare, AI models can generate synthetic medical data to simulate different treatment scenarios, helping doctors make better-informed decisions.
In finance, AI models can simulate market conditions to test investment strategies, providing valuable insights into potential outcomes.
Real-world Applications and Case Studies
Generative AI development services have been successfully implemented across various industries, demonstrating their versatility and effectiveness. Here are a few notable examples:
Healthcare:
Generative AI models have been used to create synthetic medical images, aiding in the training of radiologists and improving diagnostic accuracy. This has been particularly beneficial in identifying rare diseases where real-world data is scarce.
Finance:
Financial institutions use generative AI to detect fraudulent transactions by simulating potential fraud scenarios. This proactive approach helps in identifying and preventing fraudulent activities, ensuring the security of financial systems.
Marketing:
Businesses leverage generative AI to create personalised marketing content. AI models analyse customer data to generate targeted advertisements and recommendations, enhancing customer engagement and driving sales.
Generative AI Tools and Technologies
Overview of Available Tools
Generative AI development services leverage various tools to create advanced AI solutions. These tools include frameworks like TensorFlow and PyTorch, which provide the necessary libraries for building and training AI models.
Additionally, tools like GAN Lab and Runway ML offer user-friendly interfaces for experimenting with generative models. By using these tools, developers can streamline the creation process, ensuring efficient and accurate results.
Deep Learning Techniques
Deep learning techniques are central to generative AI. These techniques involve training neural networks on large datasets to learn complex patterns and generate new content. Key methods include Generative Adversarial Networks (GANs) and Variational Autoencoders (VAEs).
GANs use two networks—a generator and a discriminator—that work together to create realistic data. VAEs, on the other hand, encode data into a latent space and decode it back to generate new data points. These techniques enable the creation of high-quality, original content.
Image Generation and AI-Powered Image Generators
Image generation is a prominent application of generative AI. AI-powered image generators use advanced algorithms to create realistic images from scratch.
For example, GANs have been used to generate photorealistic images of faces, landscapes, and even artwork.
These generators are valuable in various industries, including entertainment, marketing, and design, where high-quality visuals are essential. By automating the image creation process, businesses can save time and resources while producing engaging content.
Challenges and Solutions in Generative AI Development
Common Obstacles in AI Development
Generative AI development services face several challenges. One major obstacle is data quality and availability.
High-quality, diverse datasets are essential for training effective AI models, but obtaining such data can be difficult. Another challenge is computational cost. Training advanced AI models requires significant computational resources, which can be expensive and time-consuming.
Additionally, ensuring model accuracy and avoiding biases is crucial. Bias in training data can lead to biased outcomes, which is particularly problematic in applications like healthcare and finance.
Finally, integrating generative AI solutions into existing systems without disrupting operations is a complex task that requires careful planning and execution.
Effective Solutions and Best Practices
Addressing these challenges requires a strategic approach. Here are some effective solutions and best practices:
Data Management: Ensure high-quality data by using data augmentation techniques and sourcing diverse datasets. Regularly update and clean the data to maintain its relevance.
Optimising Computational Resources: Use cloud-based solutions like AWS and Azure to scale computational resources as needed. This approach can help manage costs and improve efficiency.
Bias Mitigation: Implement bias detection and correction techniques during the model training phase. Use diverse datasets to ensure fair and unbiased outcomes.
Seamless Integration: Work with experienced AI development services that understand your existing systems. Develop a phased implementation plan to integrate generative AI solutions smoothly, minimising disruptions.
Choosing the Right Generative AI Development Company
Key Factors to Consider
When selecting a generative AI development company, it’s crucial to evaluate several factors to ensure you partner with the right experts.
First, assess their experience and expertise in generative AI, machine learning, and deep learning. Look for a proven track record with case studies or examples of successful projects.
Additionally, consider their technological stack and ability to integrate with your existing systems.
Ensure they use the latest tools and technologies like TensorFlow, PyTorch, and cloud platforms such as AWS or Azure. Finally, review client testimonials and industry reputation to gauge their reliability and quality of service.
Top Companies in Generative AI Development
Several companies stand out in the generative AI development space. Companies like OpenAI, known for their advancements in AI and machine learning, offer state-of-the-art generative AI solutions.
Google AI is another leader, with extensive resources and expertise in developing cutting-edge AI technologies.
Other notable mentions include Microsoft, with its Azure AI platform, and IBM, which has made significant strides in AI research and development.
These companies provide comprehensive generative AI development services, helping businesses innovate and stay competitive.
Comparing Services and Solutions
When comparing generative AI development services, consider the range and depth of solutions offered.
Look for companies that provide end-to-end services, from initial consultation and strategy development to implementation and ongoing support.
Evaluate their ability to customise solutions to fit your specific needs. For example, some companies specialise in particular industries or applications, such as healthcare, finance, or marketing.
Assess their support structure and training programs to ensure your team can effectively use and maintain the AI solutions.
How FX31 Labs Can Be Used for Generative AI Development Services
Our Approach
Client Detailed Tech and Business Assessment:
We start by understanding your specific needs, goals, and technical challenges in generative AI development. Our detailed assessment ensures we grasp your business requirements fully.
Agreement on Needs/Demand from Client:
We work closely with you to agree on the exact scope of the project, defining deliverables and the timeline. This ensures transparency and sets clear expectations.
Identification of Team Composition and Skillset:
Based on your requirements, we identify the ideal team composition. Our skilled engineers specialise in AI/ML, deep learning, and other relevant technologies, ensuring we have the right expertise for your project.
Sourcing and Hiring Tailored to Client Demand:
We source and hire top talent tailored to your specific needs. With access to world-class academicians and domain experts, we ensure the best fit for your generative AI development project.
Onboarding, Training, Managing, and Continuous Performance Evaluation:
Once the team is in place, we handle onboarding, provide necessary training, and manage the team. We also conduct continuous performance evaluations to ensure high-quality delivery.
Expertise and Technologies
Our team is proficient in a wide range of technologies essential for generative AI development:
Classical and Emerging Technologies: Algorithms, computational geometry, performance optimisation, blockchain, AI/ML/DL, IoT.
Web and CI/CD Technologies: VueJs, JavaScript, Angular/React JS, Rest APIs, Docker, AWS, Azure.
Programming Languages: C++, Java, .NET/C#, Python, R.
Data Management Technologies: SQL, Oracle, PostgreSQL, MongoDB, Hadoop, Tableau, PowerBI.
Why Choose FX31 Labs?
Choosing FX31 Labs for your generative AI development services means partnering with a team of experts dedicated to delivering high-quality solutions.
We leverage the latest technologies and methodologies to ensure your project’s success. Our commitment to continuous improvement and performance evaluation ensures we deliver results that exceed expectations.
Conclusion
FX31 Labs stands out as a premier choice for generative AI development services. Our comprehensive approach begins with a detailed assessment of your technical and business needs, ensuring we fully understand your goals and challenges.
We then tailor our team composition and skillset to meet these specific requirements, sourcing and hiring top talent from a pool of world-class academicians and domain experts.
Our expertise spans a wide range of classical and emerging technologies, including AI/ML, deep learning, and IoT, ensuring we can handle diverse project demands.
We are proficient in various programming languages and data management technologies, which allows us to provide robust and scalable solutions.