Agentic AI vs Traditional AI: Key Differences Explained for 2025
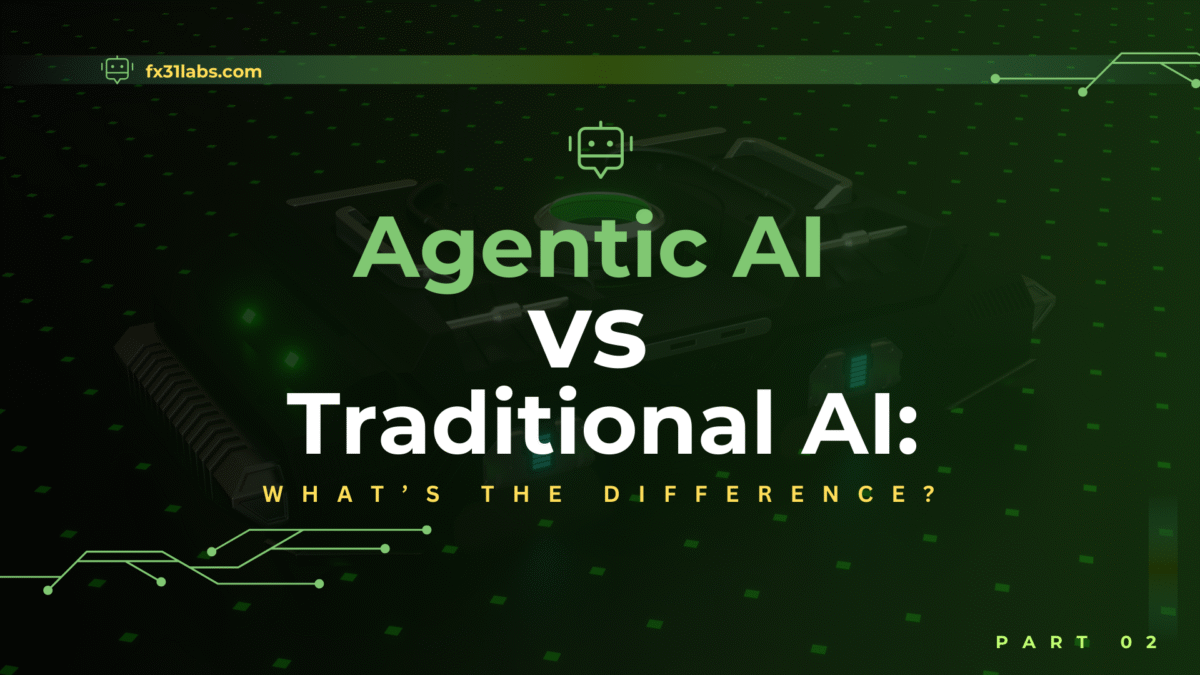
Introduction: Understanding the Shift from Traditional AI to Agentic AI
Artificial intelligence has long been a cornerstone of technological advancement, from automating routine tasks to enhancing decision-making processes across industries. However, as businesses demand increasingly sophisticated and autonomous systems, a new type of AI is emerging—Agentic AI. Unlike traditional AI, which is generally limited to predefined tasks and reactive behavior, Agentic AI introduces a level of autonomy and goal-directed problem-solving that allows systems to make decisions, learn from past interactions, and even take action on their own.
This blog aims to provide a clear comparison between Agentic AI and Traditional AI, highlighting their core differences in terms of functionality, autonomy, decision-making capabilities, and real-world applications. By the end of this post, you will understand how Agentic AI represents a leap forward in AI capabilities, offering unprecedented advantages for businesses seeking intelligent, adaptive systems.
Also Read Part 1: What Is Agentic AI?
Defining Traditional AI: Reactive, Rule-Based, and Narrow
Traditional AI refers to the AI systems that have been in use for decades, built to automate specific tasks based on rules, data patterns, or machine learning models. These systems excel in scenarios where tasks are well-defined, such as image recognition, natural language processing, or recommendation engines. However, traditional AI is limited in its ability to act beyond its predefined boundaries. It cannot initiate actions independently or adapt its behavior in response to changing conditions unless explicitly reprogrammed or retrained.
Key Characteristics of Traditional AI:
- Rule-Based or Supervised Learning: Traditional AI relies on training algorithms with large datasets to learn patterns and make predictions. For example, supervised machine learning requires labeled data to “teach” the AI how to respond correctly in different situations.
- Stateless Operation: Traditional AI systems typically do not retain memory or context beyond the current task. Once an interaction is completed, they cannot use past data or outcomes to inform future actions. This limits their ability to handle tasks that require contextual understanding or continuous learning.
- Narrow Scope: Traditional AI models are task-specific, meaning they are designed to perform well in one application (e.g., spam filters, medical diagnostic tools, or autonomous systems for specific industrial processes). Their capabilities are limited to what they’ve been specifically trained or programmed to do, and they lack the ability to pivot or operate in different domains without extensive retraining.
Real-World Examples of Traditional AI:
- Chatbots: While traditional chatbots can provide responses to customer queries, they are constrained by scripted dialogues and fixed decision trees. They cannot initiate conversations or solve problems that fall outside of their programmed scope.
- Recommendation Systems: These systems analyze user data (like browsing or purchase history) to suggest relevant products or content. They’re highly effective for personalized experiences but do not adjust or evolve in real-time based on a changing environment or new goals.
Limitations of Traditional AI:
- No Autonomy: Traditional AI requires continuous human input or predefined instructions to function. It cannot take independent actions or reorient its goals based on changing environments.
- Rigid Problem Solving: These AI systems solve problems in a linear, one-off manner, lacking the ability to adapt, replan, or self-correct in complex, multi-step tasks.
Defining Agentic AI: Autonomous, Adaptive, and Goal-Driven
Agentic AI represents the next frontier in artificial intelligence. These systems do more than just process data and respond to commands—they are capable of autonomously initiating actions, setting goals, and adapting to new circumstances. Unlike traditional AI, which is confined to narrow tasks and reactive behavior, Agentic AI is designed for goal-directed decision-making, involving complex reasoning and learning from past experiences.
Key Characteristics of Agentic AI:
- Autonomy and Goal-Setting: Agentic AI systems are self-directed and can establish their own objectives. They can analyze a given problem, set a goal, and autonomously decide the best course of action to achieve that goal. This autonomous decision-making means they can operate independently without continuous human input.
- Multi-Step Reasoning and Planning: Unlike traditional AI, which may solve problems in a direct, single-step manner, Agentic AI can break down complex problems into smaller, manageable tasks. It can create a step-by-step plan, act on it, evaluate progress, and adjust strategies as necessary. This capacity for multi-step reasoning is essential for applications like autonomous driving or dynamic supply chain management, where real-time decisions and updates are crucial.
- Memory and Contextual Awareness: One of the standout features of Agentic AI is its ability to retain context and learn over time. This memory capability allows these systems to adapt their actions based on past experiences, improving their decision-making and efficiency. In contrast, traditional AI typically starts from scratch with each new task.
- Tool Use and API Integration: Agentic AI is not limited to producing outputs—it can actively interact with external systems to execute tasks, gather information, and perform complex operations. For example, an agentic AI can query APIs, integrate data from various sources, and even initiate workflows across different platforms. This integration allows it to operate across multiple domains and accomplish tasks that traditional AI cannot.
Real-World Examples of Agentic AI:
- Autonomous Vehicles: Self-driving cars are a prime example of Agentic AI. These vehicles do not simply follow pre-programmed paths—they set driving goals, evaluate traffic conditions, calculate the best routes, and adjust their actions dynamically in response to road conditions and obstacles. They can learn from real-time feedback and improve their driving strategies over time.
- AI Personal Assistants (Advanced): Modern AI assistants, like Siri or Google Assistant, are capable of managing complex workflows. They can schedule meetings, send emails, and manage multiple tasks simultaneously. What makes them agentic is their ability to understand and act on a wide range of tasks across different platforms—like pulling up calendar entries, sending Slack messages, or interacting with external tools and APIs, all while learning from past interactions to improve their effectiveness.
Why Agentic AI is Different:
- Self-Improvement: Agentic AI doesn’t simply follow instructions—it actively learns from past actions, improves its strategies, and adapts to new information. Over time, this makes it more efficient and capable of solving more complex, real-world problems.
- True Autonomy: These systems can initiate actions, set new objectives, and perform tasks in a way that mimics human decision-making. This is a stark contrast to traditional AI, which only reacts based on inputs.
Key Dimensions of Comparison Between Agentic AI Vs Traditional AI
To truly understand the difference between Agentic AI and Traditional AI, we must look beyond surface-level tasks and explore how they fundamentally differ in architecture, capabilities, and behavior. Here are the six key dimensions that separate these two paradigms of artificial intelligence:
a. Autonomy & Initiative
- Traditional AI is reactive by nature. It only operates when prompted—whether it’s a chatbot responding to a query or a recommendation engine offering suggestions based on past clicks. It lacks the ability to initiate actions or set goals independently.
- Agentic AI, by contrast, is proactive. These systems can self-initiate actions, monitor progress toward goals, and even pivot strategies when needed. They don’t just wait for instructions—they identify what needs to be done and take action accordingly.
Example: While a traditional virtual assistant might wait for a command like “Schedule a meeting,” an agentic system might notice a scheduling conflict, suggest new times, and notify all parties—without being told.
b. Scope of Operation
- Traditional AI operates within narrow boundaries. It’s built for specific, well-defined tasks—like detecting faces in images or classifying email as spam or not spam. Its capabilities don’t extend far beyond that use case.
- Agentic AI works across multiple domains and systems, chaining tasks and decisions to complete broader workflows. It’s designed for generalization and multi-domain coordination.
Example: Instead of just analyzing support ticket sentiment, an agentic AI might also update the CRM, tag priority level, assign it to the right team, and notify the user via email.
c. Planning & Sequencing
- Traditional AI systems don’t plan—they execute a single operation at a time, often within stateless environments. They’re like advanced calculators: fast, but focused on one problem at a time.
- Agentic AI excels at multi-step reasoning. It can decompose complex goals into subtasks, sequence them logically, adjust on the fly, and replan when necessary. This gives it the ability to handle nuanced workflows that evolve over time.
Example: An agentic AI optimizing a marketing campaign could adjust audience segments, pause underperforming ads, and reallocate budgets—all autonomously and continuously.
d. Memory & Context Retention
- Traditional AI has a short memory—if it has one at all. Many systems are stateless, meaning they don’t retain information between sessions or even within extended conversations.
- Agentic AI is stateful. It maintains memory across sessions and interactions, enabling personalized and context-aware responses. It can remember past goals, mistakes, and successful strategies—crucial for improving performance over time.
Example: An agent helping with sales outreach could recall a customer’s preferences, past meetings, and outcomes—informing future engagement with nuance.
e. Tool & API Integration
- Traditional AI usually outputs results—text, classifications, predictions—but doesn’t take direct action in external systems.
- Agentic AI interacts directly with tools, APIs, and databases. It can write to files, pull data from dashboards, make web requests, or trigger workflows in SaaS platforms.
Example: Rather than suggesting “Check analytics,” an agentic AI could pull the actual metrics from Google Analytics and summarize key performance insights.
f. Adaptivity & Learning
- Traditional AI adapts slowly. It typically requires retraining or manual tuning by data scientists to improve performance or correct for drift.
- Agentic AI can adapt in near-real time. Through feedback loops and self-reflection mechanisms, it can improve its decision-making while operating—without full retraining.
Example: An agentic AI used for customer onboarding might notice drop-offs at a certain step and revise the onboarding process, all autonomously.
Also Check: OpenAI on Autonomous Agents
Real-World Examples: Traditional vs Agentic AI in Action
To help crystallize the contrast, let’s examine two familiar domains—customer support and software development—and see how traditional and agentic AI differ in real-world scenarios.
💬 Customer Support Automation
Feature | Traditional AI Support Bot | Agentic AI Support Agent |
Input Handling | Scripted Q&A, static flows | Understands context, prioritizes tasks |
Escalation | Fallback to human agent | Decides whether to escalate based on severity/history |
Action | Responds with predefined messages | Creates tickets, follows up via email, updates systems |
Example: A user reports a late delivery.
- Traditional AI replies with a canned apology.
- Agentic AI checks shipment status, sends delay notice, updates the order management system, and offers compensation if applicable.
👨💻 Software Development
Feature | Traditional Code Generator | Agentic Developer Agent |
Output | Suggests code snippets | Writes features, tests, and documentation |
Scope | Single file or block-level | Project-level goals and changes |
Action | Needs developer to review + merge | Can raise PRs, run builds, resolve minor errors |
Example:
- Traditional AI helps autocomplete a Python function.
- Agentic AI takes a Jira ticket, writes the code, tests it, updates dependencies, and opens a PR.
These use cases demonstrate that while traditional AI assists, agentic AI acts—changing how businesses scale operations and solve complex challenges.
When to Use Agentic AI vs Traditional AI
Choosing the right AI approach isn’t about which is better overall—it’s about matching the solution to the problem.
Use Traditional AI When:
- The task is narrowly defined and repetitive
- Predictability and control are paramount
- Real-time learning or goal-setting isn’t needed
Examples:
- Classifying support tickets
- Detecting spam
- Generating product recommendations
Use Agentic AI When:
- The problem requires multi-step execution
- Multiple tools, APIs, or systems need orchestration
- You need autonomy, not just automation
Examples:
- Lead nurturing in sales funnels
- Automated incident response in DevOps
- Cross-channel marketing campaign orchestration
🔄 Combine Both for a Hybrid Architecture
Many advanced AI systems are hybrid by design:
- Traditional AI models handle core tasks—image detection, classification, speech-to-text.
- Agentic AI agents orchestrate those tasks, enabling full workflows with decision-making, tool use, and iterative improvements.
This hybrid approach balances performance, governance, and agility, making it ideal for modern enterprise needs.
Implications for Businesses & Teams
As the adoption of Agentic AI accelerates, its implications ripple across team structures, infrastructure, and operational models in enterprises. Businesses looking to harness this new paradigm must adapt at both the strategic and tactical levels.
Evolving Team Structures: Rise of “Agent Ops”
Traditional AI initiatives often relied heavily on data scientists, ML engineers, and backend developers. However, Agentic AI introduces new roles like Agent Operations (Agent Ops) specialists, whose job is to monitor, debug, and fine-tune autonomous agents in real-time. These roles are akin to SREs (Site Reliability Engineers) in DevOps—focused not on building the models, but on orchestrating, maintaining, and evolving the systems that use them.
You’ll also see greater collaboration between product managers, prompt engineers, DevOps, and UX designers, ensuring agents are aligned with user goals, operational guardrails, and compliance requirements.
Infrastructure Requirements: From Models to Ecosystems
Deploying Agentic AI requires more than just a fine-tuned model. It calls for a robust infrastructure stack:
- Vector databases (e.g., Pinecone, Weaviate) for long-term memory and retrieval.
- API orchestration layers to allow agents to call external services.
- Observability & analytics tools to log agent decisions, errors, and outcomes.
- Tool repositories & plugins to enable dynamic task execution (e.g., search, database queries, emailing, scheduling).
Businesses need to think in terms of agent ecosystems, not just isolated models.
Governance, Safety & Compliance
With autonomy comes risk. Agentic AI systems that can act and learn require strong governance:
- Human-in-the-loop systems to provide approvals at critical junctions.
- Audit trails to trace decision paths for compliance.
- Ethical AI checklists to prevent biased or harmful actions.
- Fail-safe triggers to shut down agents when they breach boundaries.
Organizations must build governance frameworks that are proactive, not reactive, to avoid real-world consequences.
ROI & Efficiency Gains
The potential payoff is significant:
- 24/7 productivity without human fatigue.
- Multi-domain coverage across departments (e.g., sales, support, R&D).
- Faster turnaround on repetitive or multi-step workflows.
- Lower operational costs by reducing manual task dependencies.
Companies leveraging Agentic AI effectively can see a 10x improvement in operational throughput, especially in knowledge-heavy functions like legal, finance, and customer support.
Future Outlook
The trajectory of AI is clear: toward greater autonomy, collaboration, and orchestration. Let’s explore what the future holds for Agentic AI.
Multi-Agent Systems Will Become the Norm
Just as humans work in teams, AI agents will increasingly operate in multi-agent environments—coordinating with other agents specialized in different tasks. For instance, a product research agent may collect data, a legal agent may review compliance, and a copywriting agent may generate messaging—all working in sync.
This model unlocks modular intelligence, where each agent plays a specific role in a broader workflow.
Emergence of Agent Marketplaces
Expect to see the rise of agent marketplaces and tool ecosystems. Developers will create and monetize agents that can be plugged into larger systems. Think of it as the next iteration of app stores—where instead of apps, you purchase and deploy intelligent agents tailored to specific use cases (e.g., bookkeeping, SEO audits, code review).
Enterprises may even have internal agent libraries for proprietary use, trained on domain-specific data and tools.
Standardization & Orchestration Platforms
Currently, deploying agentic systems is highly bespoke. But that’s changing fast. Platforms like LangChain, AutoGen, and CrewAI are building standardized frameworks for:
- Agent-to-agent communication
- Goal decomposition and task scheduling
- Real-time memory and state management
The rise of AI orchestration platforms will make building agentic systems as modular and scalable as traditional software engineering.
Risks to Watch
While promising, agentic systems come with challenges:
- Hallucinations can escalate due to autonomy.
- Over-automation may lead to errors in edge cases.
- Security risks arise if agents manipulate sensitive systems or data.
The future will require guardrails as a first-class citizen in any agent architecture.
Conclusion & Next Steps
As AI continues its march toward autonomy, the distinction between traditional AI and agentic AI is more than academic—it shapes how we build, deploy, and interact with intelligent systems.
Summary of Key Differences
- Traditional AI is reactive, narrow, and stateless. It excels at single-turn tasks like classification or text generation.
- Agentic AI is proactive, goal-driven, and stateful. It shines in multi-step workflows, tool use, and autonomous decision-making.
When to Use What
- Use traditional AI for high-volume, low-context operations (e.g., fraud detection, email filtering).
- Use agentic AI for complex, dynamic tasks that require chaining tools, adapting to new information, or operating over time (e.g., onboarding workflows, content production, automated R&D).
What’s Next?
The next blog in this series—“Why Agentic AI Matters in 2025 (And Beyond)”.
Stay tuned as we continue decoding the future of intelligent automation—and how your business can lead the charge.